In today’s fast-paced world, the importance of early and accurate diagnosis of skin conditions, including skin cancer, cannot be overstated. However, the tools traditionally used for these diagnostics are often out of reach for the average consumer due to their high costs and complexity. This can result in late treatments and lost lives.
Thanks to our partnership with David from @xleaks7, we spotted a new Google patent that will forever transform skin diagnostics.
The patent presents a groundbreaking solution that aims to democratize skin health monitoring by leveraging the technology already present in many consumer devices, such as smartphones.
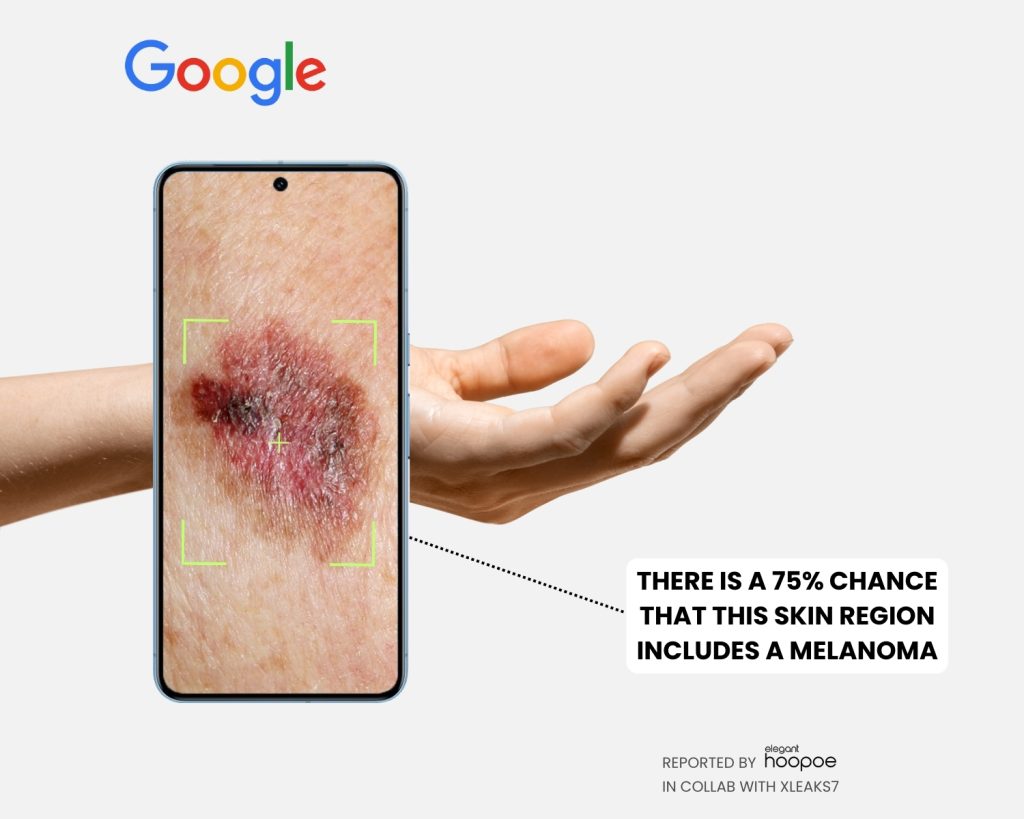
Your Personal Diagnostic Tool for Skin
This whole time you had such a powerful skin cancer diagnostic tool right in your pocket and you had no idea! With 3D ultrasonic sensor technology, your own cellphone can be your on-call skin specialist. It just takes a quick scan and you can have all the answers about your skin health. Imagine the relief of knowing your skin is fine or the peace of mind that comes from identifying a potential issue early. Either way, it’ll improve your life quality tremendously. Embrace this cutting-edge tech and let it keep you safer than ever with early detection of any skin condition.
The Problem the Patent is Going to Solve
In 2021, Google launched a trial of its “dermatology assist tool”, which can spot skin, hair, and nail conditions based on images uploaded by patients.
The app has even been awarded a CE mark in Europe.
However, the tech giant has been developing health diagnostic technology since then, and soon, we will have a more accurate tool that will democratize skin health monitoring and save lives.
The patent aims to improve the early detection and diagnosis of skin conditions, particularly skin cancer, using advanced technology.
Instead of using only images, the patent describes a method and system that uses three-dimensional (3D) sonic sensors to gather volumetric data about skin lesions, which are then analyzed using machine learning models to identify potential skin cancer conditions.
The diagnostic results, including confidence levels, are displayed on the computing device for further clinical evaluation.
The technology will reduce the load on traditional diagnostic tools for skin conditions, such as optical coherence tomography (OCT) and ultrasonography, which are typically expensive, bulky, and require specialized training to operate, and will allow an average person for easy regular check-ups.
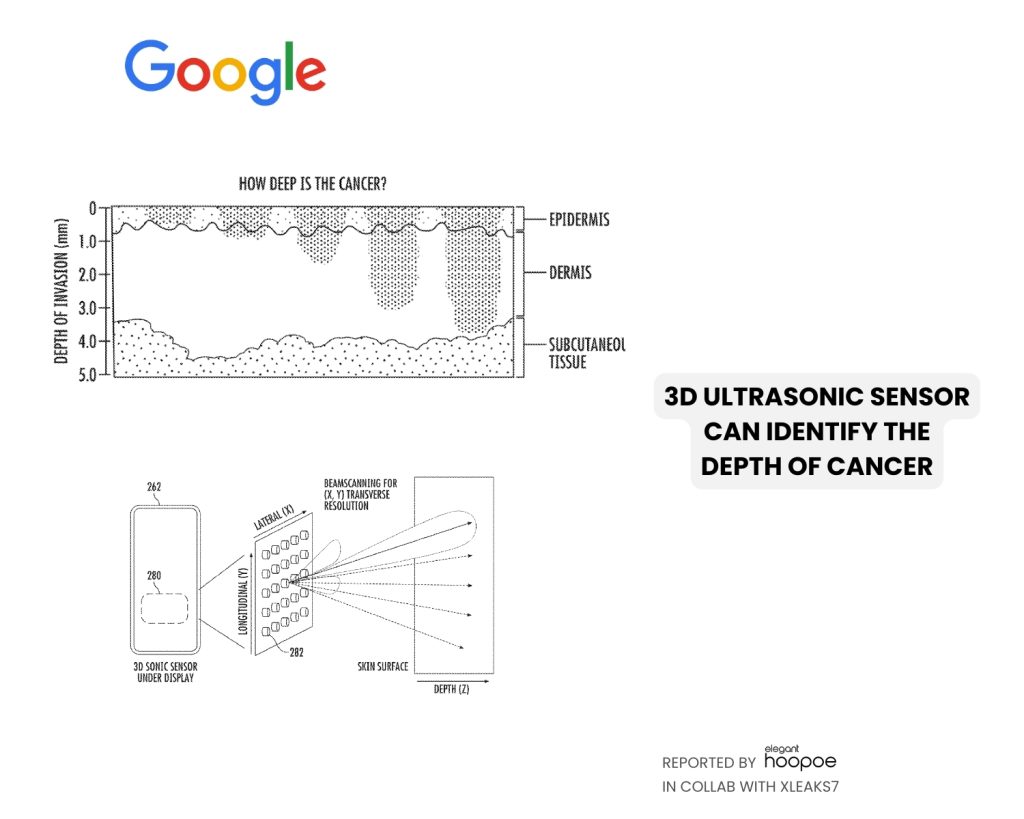
Key Points
- User Interface for Diagnosis: The system uses a smartphone to display diagnostic outputs, showing results of skin cancer analysis from a scanned lesion.
- Machine Learning Integration: The diagnostic system employs machine learning to identify skin cancer features based on volumetric data from ultrasonic pulses.
- Depth Analysis for Accuracy: Unlike surface imaging, this system analyzes the depth of skin lesions, which is crucial for distinguishing between benign and malignant conditions.
- 3D Ultrasonic Sensor: A 3D ultrasonic sensor located under the smartphone display captures detailed images of skin lesions by resolving depth information through back-reflected ultrasonic pulses.
- Imaging Technology: Ultrasonography is used to penetrate dermal layers, providing more accurate clinical data compared to traditional surface images.
- Training and Deployment: The machine learning model is trained with high-resolution sensor data and annotated features to build a dictionary of skin cancer characteristics, which is then used for diagnosing new cases.
- User Authentication: The system can also authenticate users via fingerprint scanning, using the same 3D ultrasonic sensor technology.
- Confidence Scoring: The system provides a confidence score for each diagnosis, indicating the reliability of the identified skin condition.
- Clinical Evaluation: The diagnostic process and results are designed to be transparent, allowing clinicians to review and evaluate the data and underlying diagnostic reasoning for informed decision-making.
Conclusion: What to Expect in the Future
The integration of machine learning with 3D sonic sensor technology for skin condition diagnostics is poised to transform healthcare. We can expect improved accuracy in early detection, widespread adoption in consumer devices, enhanced user personalization, and potential expansion to other medical fields. This innovation will likely lead to more accessible, precise, and effective healthcare solutions, revolutionizing the way we monitor and treat skin conditions and beyond.
NOTE TO EDITORS: The text and visuals of this article are the intellectual property of Elegant Hoopoe. If you want to share the content, please give a proper clickable credit. Thanks for understanding.